The demand for energy to power AI is increasing rapidly, with multi-billion-dollar investments on the horizon for nuclear-powered data centers. After months of public discussion, the governor of California vetoed the SB 1047 bill while LAION won a huge copyright infringement case in the German court. Join us for an overview of the latest news in AI R&D and a curated list of the month's top 10 trending research papers.
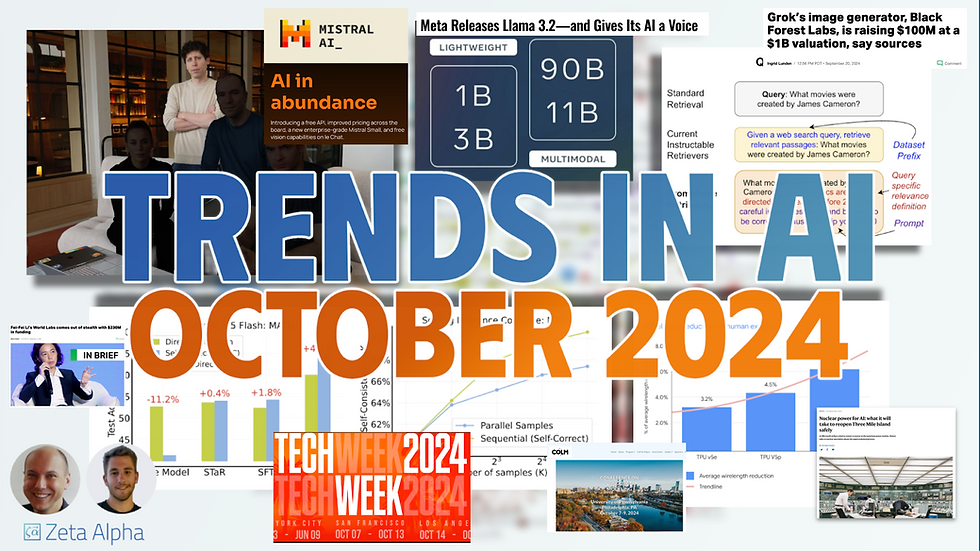
News Articles
Model Releases
Trending AI papers for October 2024
[1] Making Text Embedders Few-Shot Learners - C. Li et al. (BAAI) - 23 September 2024
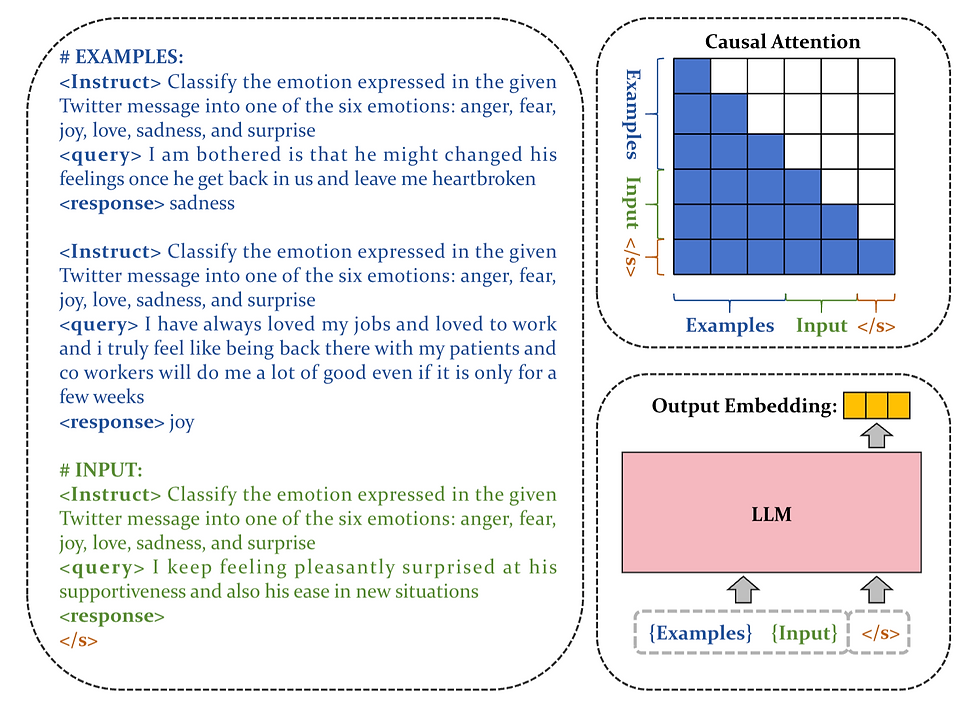
→ In-Context Learning (ICL) for embeddings. bge-en-icl uses few-shot examples to contextualize the embedding generation during query time.
🤔 Why? ICL makes LLM-based encoders more adaptable by providing information about the retrieval task during inference.
💡 Key Findings:
SOTA on MTEB & AIR-Bench.
The model performs well both on zero-shot & few-shot setups.
The report contains ablations on architectural & design choices.
[2] Promptriever: Instruction-Trained Retrievers Can Be Prompted Like Language Models - O. Weller et al. (JHU, Samaya AI) - 17 September 2024
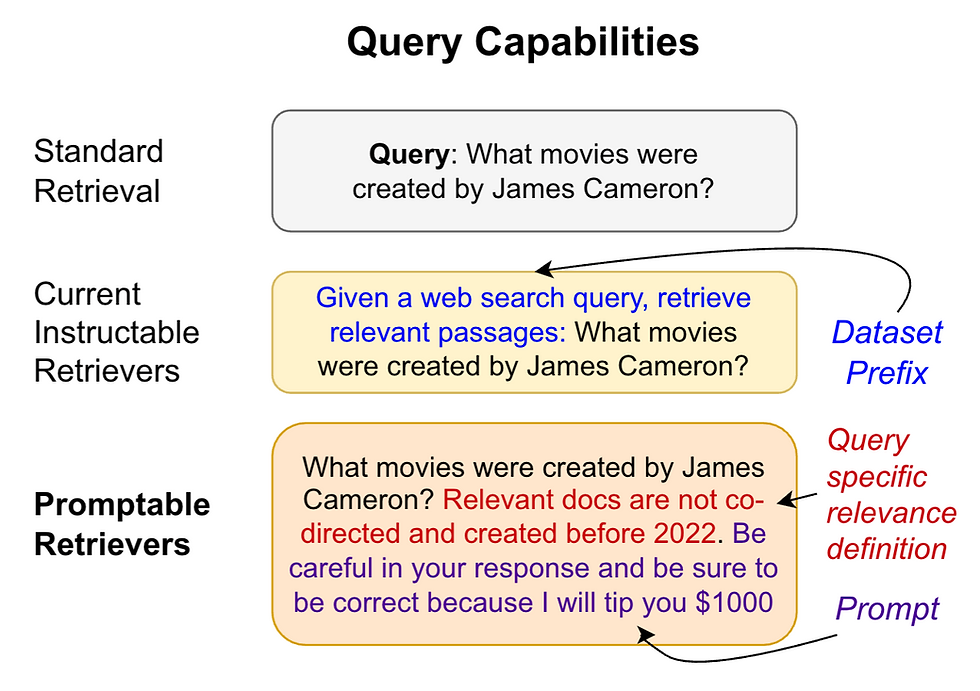
→ Promptriever: a method for embedding models to follow instance-level instructions to better match queries to relevant passages.
🤔 Why? When LLMs are finetuned for retrieval embeddings they typically lose their instruction following capability. Earlier works have only generated task level instructions.
💡 Key Results:
A newly curated dataset from MS MARCO, enriched with instance-level instructions.
Promptriever achieves performance improvement compared to RePLLaMA
[3] Contextual Document Embeddings - Morris & Rush (Cornell University) - 03 October 2024
→ CDE changes the embedding of documents and queries based on similar documents, to adapt embeddings to particular collections.
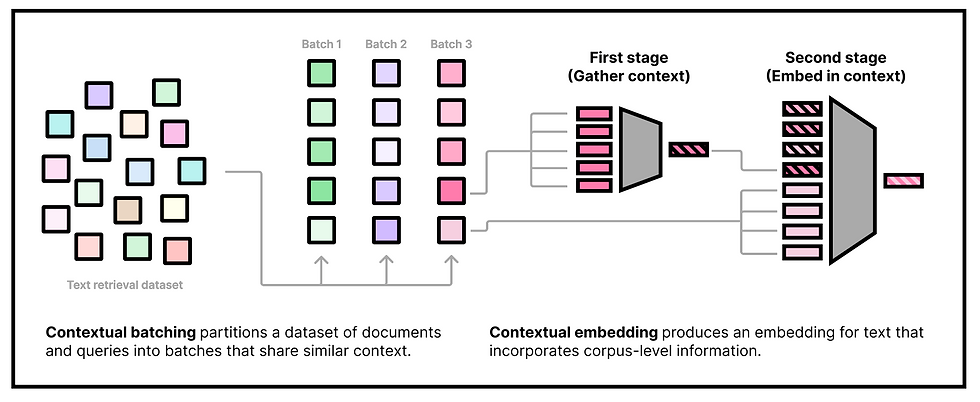
🤔 Why? Bi-encoder embeddings do not have access to collection statistics like IDF which is a problem in out-of-domain settings.
💡 Key Findings:
CDE has similarities to pseudo relevance feedback in IR, but also acts at indexing time on the document representations.
There are also connections to hard negative mining, but this only works at indexing time.
[4] Programming Every Example: Lifting Pre-training Data Quality like Experts at Scale - F. Zhou et al. (SJTU, GAIR) - 25 September 2024
→ Programming Every Example (ProX): a data refinement framework where small language models operate on a training corpus. Logical next step in using LMs as filters for low-quality data – why not also use LMs to improve them?
🤔 Why? It’s an alternative to heuristics-based data processing techniques, leading to better quality without human intervention.
💡 Key Findings:
When training on refined data:
Up to 6.2% improvement in performance.
Up to 30x fewer training steps required.
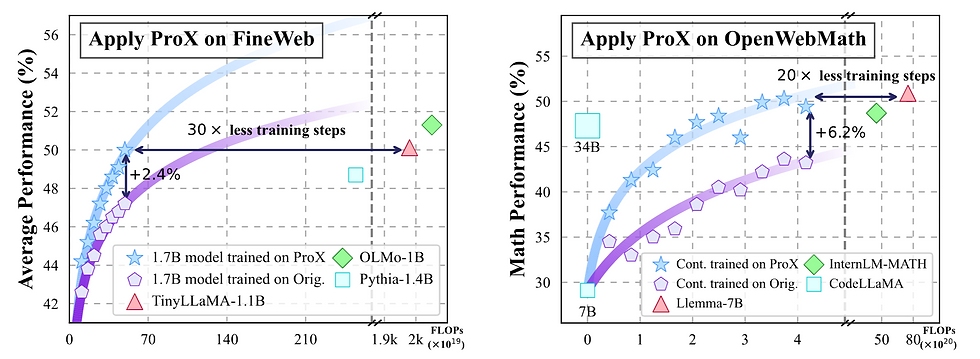
[5] Training Language Models to Self-Correct via Reinforcement Learning - A. Kumar et al. (Google DeepMind) - 19 September 2024
→ SCoRe is a new multi-stage RL framework to train LLM’s to recover from errors.
🤔 Why? Using more inference time compute, we may leverage the knowledge in the model to refine and correct first pass answers. However, simple prompting leads to degradation instead.
💡 Key Findings:
Supervised finetuning is not effective to learn self-correction behaviors, due to distribution shift and amplification of bias and ineffective pathological behaviors.
Comparison on MATH and HumanEval shows improvements on accuracy at the first and second attempt over previous models.
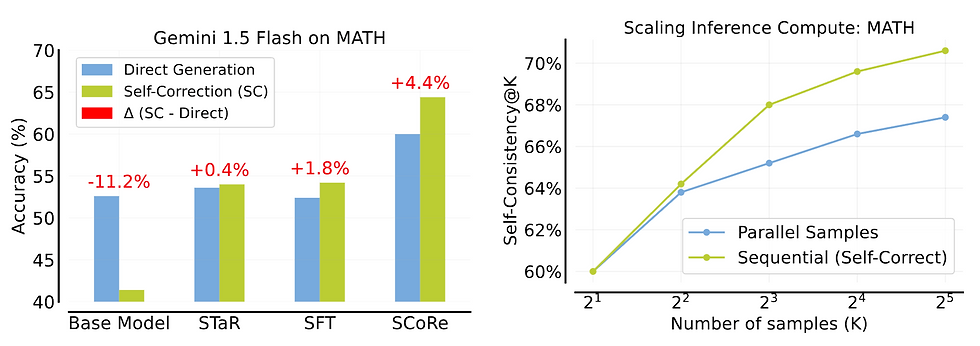
[6] MIO: A Foundation Model on Multimodal Tokens - Z. Wang et al. (BUAA, 01.AI) - 26 September 2024
→ MIO (Multimodal Input and Output): foundation model that unifies text, image, speech & video.
It is the first open-source* model capable of any-to-any multimodal interleaved generation.
It involves four stages of training: (i) alignment pre-training, (ii) interleaved pre-training, (iii) speech-enhanced pre-training, (iv) supervised multimodal fine-tuning
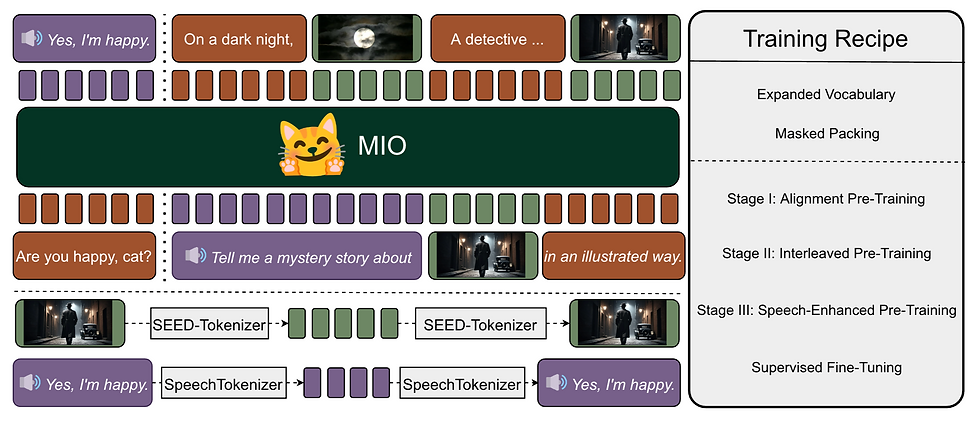
🤔 Why? It enables tasks such as interleaved video-text generation, chain-of-visual-thought reasoning, and visual guideline generation.
💡 Key Findings:
Competitive performance on:
Image tasks: captioning, visual QA
Speech tasks: TTS (& ASR)
Video tasks: video QA, video generation
[7] Imagine yourself: Tuning-Free Personalized Image Generation - Z. He et al. (Meta) - 20 September 2024
→ Imagine yourself: a tuning-free personalized image generation model that relies entirely on text prompting.
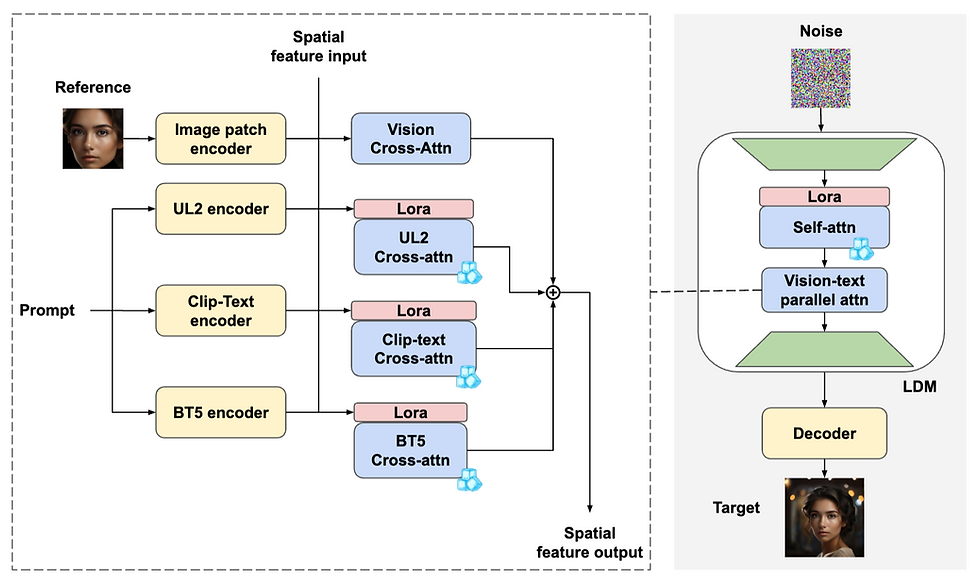
🤔 Why? This approach is much more efficient when scaling to a large number of users, as there is no need for user-specific fine-tuning.
💡 Key Findings:
Human evaluation on three axes: visual appeal, identity preservation, and prompt alignment.
31.6% win rate in visual appeal (vs 11.5% for the SOTA control-based model).
5.5% win rate in identity preservation (vs 3.8% for the SOTA adapter-based model).
46.3% win rate in prompt alignment (vs 1.2% for the SOTA control-based model).
[8] Moshi: a speech-text foundation model for real-time dialogue - A. Défossez et al. (Kyutai) - 18 September 2024

→ Moshi is an open source model for speech and text allowing full-duplex dialogue on your PC.
🤔 Why? Traditional speech systems have multiple modules that introduce high latency, and lose non-linguistic speech information.
💡 Key Findings:
Moshi reduces latency from ~2s to ~160ms, allowing real-time interactions.
Incorporates non-linguistic information such as emotions and interjections, for more natural conversations.
Overlapping speech and natural interruptions, like in human dialogues.
[9] LLaVA-3D: A Simple yet Effective Pathway to Empowering LMMs with 3D-awareness - C. Zhu et al. (HKU) - 26 September 2024
→ LLaVA-3D: extending multimodal models to 3D scene understanding with 3D patches, by integrating spatial info to 2D visual features.
🤔 Why? It is a lightweight approach to enabling 3D scene understanding without training from scratch or adding architectural modifications.
💡 Key Findings:
SOTA on 3D QA tasks as well as 3D dense captioning tasks.
Maintains performance in 2D tasks.
Faster in both inference and training compared to existing 3D models.
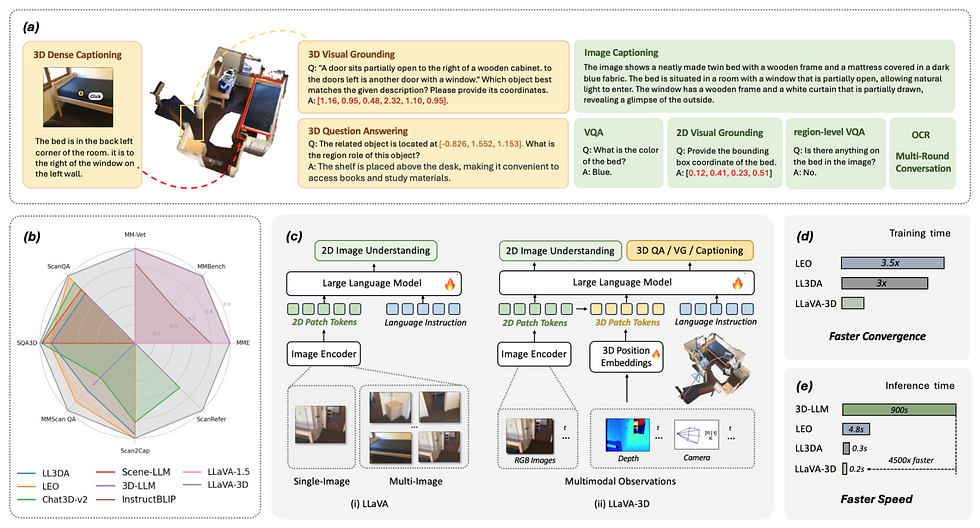
[10] LLMs Still Can't Plan; Can LRMs? A Preliminary Evaluation of OpenAI's o1 on PlanBench - K. Valmeekam et al. (ASU) - 20 September 2024
→ Evaluation of Large Language Models (LLMs) and Large Reasoning Models (LRMs) on PlanBench.
🤔 Why? Recent emphasis on reasoning in OpenAI models suggests important progress on planning tasks.
💡 Key Findings:
o1 Performance: OpenAI's o1 model shows significant improvement but still falls short of full saturation on the benchmark.
Raises important questions about the accuracy, efficiency, and guarantees of deploying such systems.
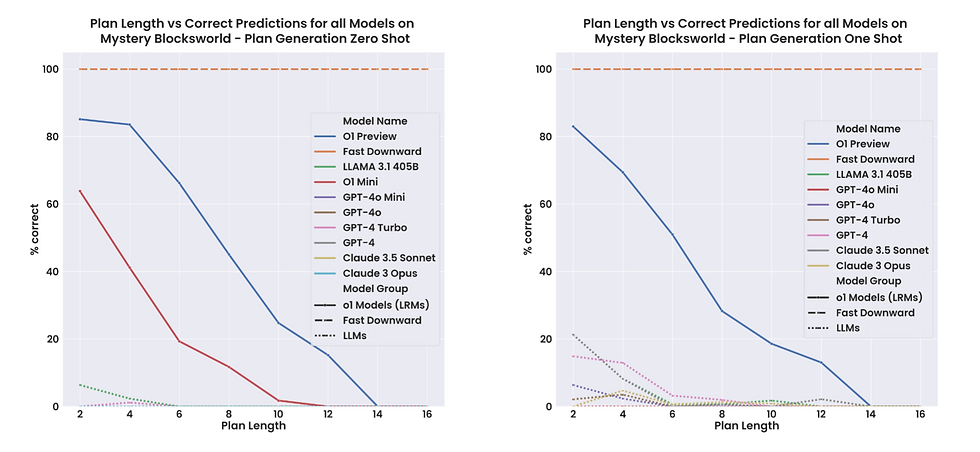
And a few runner-ups:
MM1.5: Methods, Analysis & Insights from Multimodal LLM Fine-tuning - H. Zhang et al. (Apple) - 30 September 2024
Emu3: Next-Token Prediction is All You Need - X. Wang et al. (BAAI) - 27 September 2024
OmniGen: Unified Image Generation - S. Xiao et al. (BAAI) - 17 September 2024
LLMs + Persona-Plug = Personalized LLMs - J. Liu et al. (RUC-GSAI) - 18 September 2024
Don't Use LLMs to Make Relevance Judgments - I. Soboroff (NIST) - 23 September 2024
GRIN: GRadient-INformed MoE - L. Liu et al. (Microsoft) - 18 September 2024
NVLM: Open Frontier-Class Multimodal LLMs - W. Dai et al. (NVIDIA) - 17 September 2024
The Perfect Blend: Redefining RLHF with Mixture of Judges - T. Xu et al. (Meta) - 30 September 2024
You can find an annotated collection of these papers (+ more that didn't make the cut) in Zeta Alpha, allowing you to easily discover relevant literature and dive deeper into any topic that interests you.
Here is a 3-minute preview of the papers in our top-10 list:
The full recording of our latest Trends in AI episode is available on our YouTube, covering all of the papers in depth. Sign up to join us live for the next edition in November. Until then, enjoy discovery!
Comentários