Zeta Alpha's Trends in AI webinar kicked off the new year by looking at the recent progress in AI and we extrapolate these trends to predict where we think things are headed for 2025. Read on if you want to understand the key developments impacting AI in the next 12 months and get up to speed quickly.
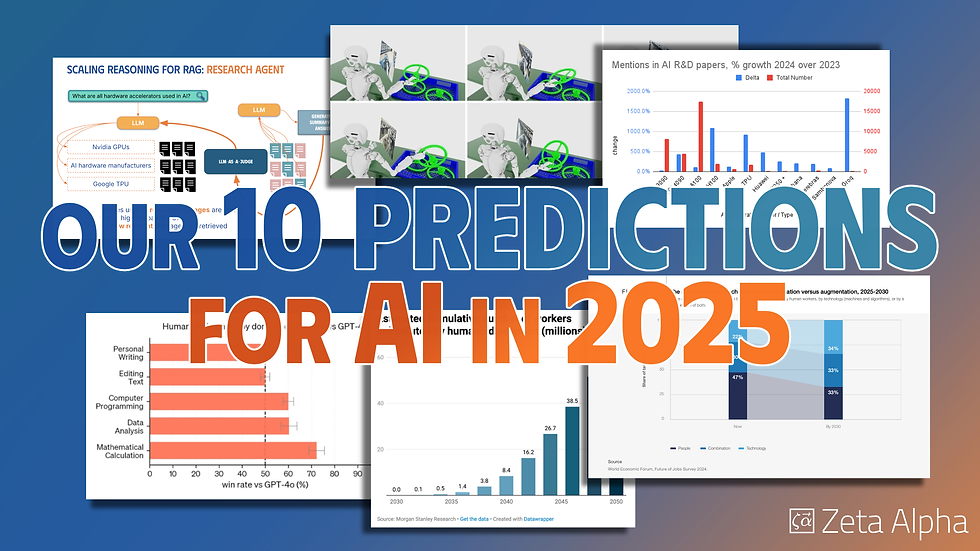
Catch us live for the next edition of our webinar for all the latest news and developments in AI:
Without further ado: Our 10 Predictions for AI in 2025:
Inference-time compute scaling will dominate AI progress.
![Figure 1: o1's performance on AIME improves with more test-time compute. [Source]](https://static.wixstatic.com/media/de4c56_77056208819d4d72ba3ba30480a50027~mv2.png/v1/fill/w_980,h_1082,al_c,q_90,usm_0.66_1.00_0.01,enc_avif,quality_auto/de4c56_77056208819d4d72ba3ba30480a50027~mv2.png)
The trend is shifting from the massive compute used for training AI models to optimizing how they perform during inference. Using more tokens at inference time seems like the most straightforward way of improving the performance of large language models (Fig. 1). This is supported by ongoing research that seeks to replicate models such as OpenAI's o1 (and recently, o3), as they've been the only models to break the ARC-AGI benchmark (Fig. 2). While these models may not show across-the-board improvements, they excel in areas like mathematical reasoning and code generation (Fig. 3). Interestingly, AI regulation so far has been based on training compute, whereas the industry is rapidly moving towards inference time compute. Already, we see smaller labs trying to get in on the action by distilling models similar to o1, either by training on reasoning traces or trying to replicate its reinforcement learning-based training setup. Notably, the recently released DeepSeek-R1 model is alleged to be causing panic in the large industrial AI labs as it was trained on a budget of only $6M on a relatively modest GPU cluster, while matching the performance of OpenAI's o1 model.
![Figure 2: o3 (tuned) beats the average human on the ARC-AGI benchmark. [Source]](https://static.wixstatic.com/media/de4c56_ca177b91fc02435f8b97f2acae4ac5ae~mv2.png/v1/fill/w_980,h_551,al_c,q_90,usm_0.66_1.00_0.01,enc_avif,quality_auto/de4c56_ca177b91fc02435f8b97f2acae4ac5ae~mv2.png)
![Figure 3: o1-preview isn't always preferred by humans over GPT-4o. [Source]](https://static.wixstatic.com/media/de4c56_f9df31312cb444438b02b13356436ec4~mv2.png/v1/fill/w_980,h_588,al_c,q_90,usm_0.66_1.00_0.01,enc_avif,quality_auto/de4c56_f9df31312cb444438b02b13356436ec4~mv2.png)
The compute market for inference will break NVIDIA’s dominance.
The shift towards inference time reasoning presents a new opportunity to disrupt Nvidia's stronghold. The appeal of running models on cheaper and more efficient hardware is expected to grow, especially with the increasing prevalence of edge devices. Companies like AMD and Google, with their Instinct Series GPUs and Trillium TPUs respectively, are well-positioned to eat into Nvidia's market share. As the cost of inference will become a critical factor, the high profit margins that Nvidia currently enjoys will not be sustainable. A recent analysis showed that AMD's hardware offers better flops performance, memory capacity, and a lower price compared to Nvidia (Fig. 4). Our internal report of compute accelerators in research papers also shows that the popularity of different hardware is also shifting, with TPUs and other competitors experiencing rapid growth (Fig. 5).
![Figure 4: AMD's MI300X has higher base specifications than the Nvidia H series. [Source]](https://static.wixstatic.com/media/de4c56_5474e12e76664bd0951dd0d481651d2b~mv2.png/v1/fill/w_980,h_488,al_c,q_90,usm_0.66_1.00_0.01,enc_avif,quality_auto/de4c56_5474e12e76664bd0951dd0d481651d2b~mv2.png)
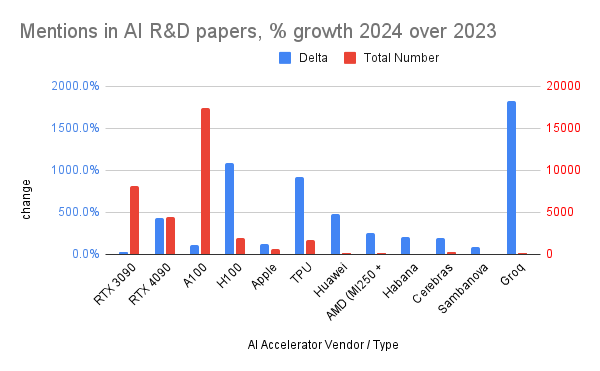
Enterprise AI systems will be based on agentic RAG.
Companies deploying Gen AI systems tend to prioritize faithfulness and accuracy, focusing on trustworthy sources and grounding answers in the source documents with references. Agents are increasingly playing a role in creating better answers through dynamic information retrieval, going beyond strict output definitions. Recently, we encountered a benchmark showing that agentic behavior helps across the board, such as in question answering and math tasks (Fig. 6). This is something we truly believe in at Zeta Alpha, as evidenced by our Research Agent release in November (read more here!), which is built on top of a well-balanced interplay between multiple LLM based agents that plan search queries to explore different aspects of the topic, evaluate the relevance of search results, and integrate information from documents into a coherent and fact-based research report.
![Figure 6: Agentic inference improves overall performance for tasks like question answering and math. [Source]](https://static.wixstatic.com/media/de4c56_c1dae2f2fc4b440a9a1e990eb40ad8eb~mv2.png/v1/fill/w_980,h_380,al_c,q_90,usm_0.66_1.00_0.01,enc_avif,quality_auto/de4c56_c1dae2f2fc4b440a9a1e990eb40ad8eb~mv2.png)
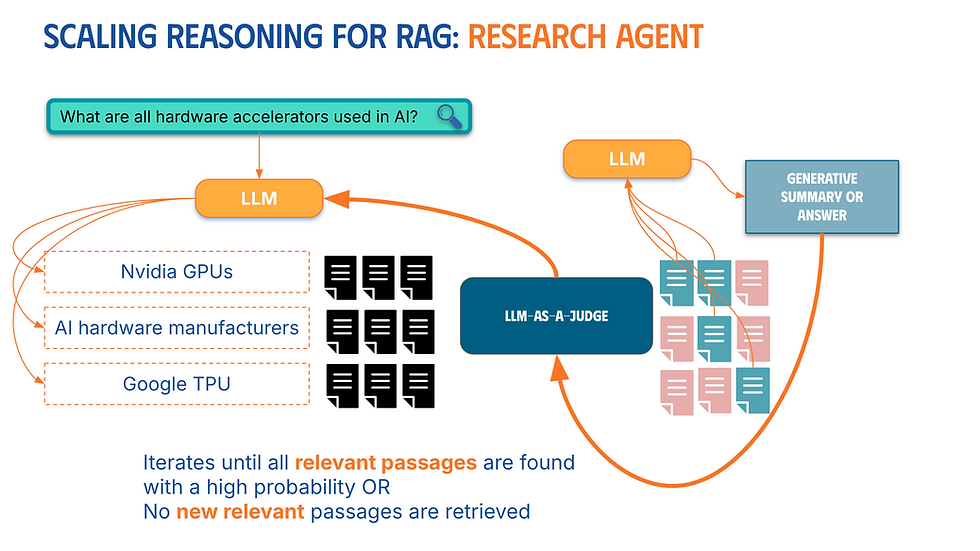
AI agents will have a measurable impact on the labor market.
Some companies, like Salesforce, have stated that they have enough software engineers, and they foresee AI agents filling any missing positions. Mark Zuckerberg also believes that AI will replace mid-level software engineers. The recent World Economic Forum Future of Jobs Report predicts a significant increase in work done by AI, which will have a corresponding impact on the labor market (Fig. 8). All these recent developments hint that the workforce as we know it is going to change in the coming years, with less critical positions being substituted, or enhanced, with AI agents.
![Figure 8: WEF predicts one third of the tasks to be completed by AI, and one third collaboratively. [Source]](https://static.wixstatic.com/media/de4c56_89bb8c96df804370b8cc1cd2702bb313~mv2.png/v1/fill/w_980,h_581,al_c,q_90,usm_0.66_1.00_0.01,enc_avif,quality_auto/de4c56_89bb8c96df804370b8cc1cd2702bb313~mv2.png)
Multimodal search will be the new normal by the end of 2025.
Vision-based search, using models like DSE and ColPali, will become the standard for searching both textual and visual documents. This paradigm greatly simplifies the required pre-processing pipelines by creating embeddings directly from visual data. We are already seeing that existing benchmarks for visually rich documents like ViDoRe are being rapidly saturated (Fig. 9). In any case, we anticipate a lot of the Neural IR research to be focusing on this new approach, as we are seeing a growing number search technology vendors, including Zeta Alpha to switch to this new paradigm because of importnant real-world applications on many data modalities, such as figures and tables, visually information-rich pages like presentation slides, etc.
![Figure 9: The ViDoRe benchmark seems to be saturating, with the SOTA reaching a score of 90 pts. [Source]](https://static.wixstatic.com/media/de4c56_e119e4b250a54d8ab67f592bd472e643~mv2.png/v1/fill/w_980,h_539,al_c,q_90,usm_0.66_1.00_0.01,enc_avif,quality_auto/de4c56_e119e4b250a54d8ab67f592bd472e643~mv2.png)
2025 will be the year of World (Foundation) Models.
Moving beyond just understanding text and images, we are observing a lot of traction around developing AI that can understand the world, including physics and how objects interact. This includes creating models that can be used to control robots, which is also referred to as embodiment. Nvidia's Cosmos model, which was recently open-sourced, is an example of this type of world model (Fig. 10). The convergence of gaming engines and video generation into this field is also noteworthy as all of these areas must have a robust physics simulation to function properly.
![Figure 10: NVIDIA Cosmos can be fine-tuned for physical control of embodied systems. [Source]](https://static.wixstatic.com/media/de4c56_4350b2e3512f4a1688da21f5e76484d4~mv2.jpg/v1/fill/w_980,h_367,al_c,q_80,usm_0.66_1.00_0.01,enc_avif,quality_auto/de4c56_4350b2e3512f4a1688da21f5e76484d4~mv2.jpg)
Humanoid robots will remain hype (other forms will be practical).
This might be a controversial take, but we predict that fewer than 10,000 humanoid robots will be sold by the end of 2025 (and we think 10K is already pushing it), indicating continued hype without significant real-world impact. The Tesla robot recently made the news with a negative connotation, as instead of being autonomous it turned out that it was teleoperated by humans behind the scenes. A recent study from Morgan Stanley Research estimated the number of workers substituted by humanoid roboids to remain below 0.1% until 2032 (Fig. 11). While we anticipate that there will be considerable investment, success is anything but certain. On a positive note, however, we expect that other form factors, like dog robots, will see more progress due to their versatility and practicality. In particular the Unitree demo video that has recently been released is stunning if real.
![Figure 11: Humanoid robots are decades away from replacing workers at scale. [Source]](https://static.wixstatic.com/media/de4c56_c1e3ad2e4551468c9a0375bae1335a8c~mv2.png/v1/fill/w_980,h_806,al_c,q_90,usm_0.66_1.00_0.01,enc_avif,quality_auto/de4c56_c1e3ad2e4551468c9a0375bae1335a8c~mv2.png)
Quantum AI computers are only scientifically ‘Interesting’ for 2025.
Quantum computing will continue to be of great interest to the scientific community, but we do not expect it to have any practical applications for AI anytime soon. Notably, Jensen Wang from Nvidia recently suggested that this tech is still 10-20 years away from being useful in production. If we were to take this at face value, it would be safe to say that for 2025, AI development will mostly continue to rely on current hardware and algorithms. Although companies like D-Wave already have solutions for interesting optimization problems running on quantum hardware, and Google, NVIDIA, IBM and many others are massively investing in quantum hardware progress with significant breakthroughs, like the recent Willow chip, the scale of these systems is nowhere near the requirements of running large AI models with billions of parameters that currently dominate the field. Unless someone suddenly has a real bright new idea, of course...
No AI-powered drone swarms deciding war outcomes.
Despite the use of AI in drones and robots for battles that are happening this very day, for which the war Ukraine is proving a big laboratory, military leaders still consider the technology not mature enough to be able to confidently deploy autonomous swarms in battle. The reason is simple; soldiers don't trust fully autonomous drones, and the risks of massive friendly fire, or edge case failures in defense are simply too high. Despite advancements in general AI capabilities, human oversight is still needed when it comes to the use of such technology in conflicts, so for the foreseeable future, we only see AI as an assistive copilot rather than a decisive factor that can single-handedly influence the outcome of a battle.
The major global (tech) conflict will be between the US and the EU.
Last but not least, we are observing a major conflict brewing between the US and the EU over tech regulation, particularly concerning AI. The US export controls on AI technology recently proposed by President Biden, as well as the push for domestic AI dominance, are key points of contention. The EU, known for its strong regulatory stance, is expected to push back and hold its ground. We expect this disagreement to grow, and to have a noticeable impact on the global tech landscape. We might be biased, but we find that the EU is more likely to prevail due to its thorough regulatory framework that is already in place, plus the fact that big tech realistically cannot afford to lose access to the European market. At the same time, China is making very rapid progress in open source AI, which could make US export controls less effective and give the EU alternative new source of AI technology.
Watch the full recording of our January edition of the Trends in AI webinar to get the bigger picture behind these predictions, along with relevant news headlines, model releases, and research papers that we didn't get to cover in this blog post:
We will revisit the success of our crystal ball at the end of the year, and keep you informed on AI progress in the mean time. Sign up for Zeta Alpha to stay in-the-know and receive your own personalized AI recommendations every day.
Until next time, and Enjoy Discovery!
Comments