Must read: the 100 most cited AI papers in 2022
- Jakub Zavrel
- Mar 2, 2023
- 6 min read
Updated: Mar 8, 2023
Who Is publishing the most Impactful AI research right now? With the breakneck pace of innovation in AI, it is crucial to pick up some signal as soon as possible. No one has the time to read everything, but these 100 papers are sure to bend the road as to where our AI technology is going. The real test of impact of R&D teams is of course how the technology appears in products, and OpenAI shook the world by releasing ChatGPT at the end of November 2022, following fast on their March 2022 paper “Training language models to follow instructions with human feedback”. Such fast product adoption is rare, so to see a bit further, we look at a classic academic metric: the number of citations. A detailed analysis of the 100 most cited papers per year, for 2022, 2021, and 2020 allows us to draw some early conclusions. The United States and Google still dominate, and DeepMind has had a stellar year of success, but given its volume of output, OpenAI is really in a league of its own both in product impact, and in research that becomes quickly and broadly cited. The full top-100 list for 2022 is included below in this post.
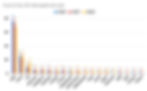
Using data from the Zeta Alpha platform combined with careful human curation (more about methodology below), we've gathered the top cited papers in AI from 2022, 2021, and 2020, and analyzed authors' affiliations, and country. This allows us to rank these by R&D impact rather than pure publication volume.
What are some of these top papers we're talking about?
But before we dive into the numbers, let's get a sense of what papers we're talking about: the blockbusters from these past 3 years. You'll probably recognize a few of them!
2022
1️⃣ AlphaFold Protein Structure Database: massively expanding the structural coverage of protein-sequence space with high-accuracy models -> (From DeepMind, 1372 citations) Using AlphaFold to augment protein structure database coverage.
2️⃣ ColabFold: making protein folding accessible to all -> (From multiple institutions, 1162 citations) An open-source and efficient protein folding model.
3️⃣ Hierarchical Text-Conditional Image Generation with CLIP Latents -> (From OpenAI, 718 citations) DALL·E 2, complex prompted image generation that left most in awe.
4️⃣ A ConvNet for the 2020s -> (From Meta and UC Berkeley, 690 citations) A successful modernization of CNNs at a time of boom for Transformers in Computer Vision.
5️⃣ PaLM: Scaling Language Modeling with Pathways -> (From Google, 452 citations) Google's mammoth 540B Large Language Model, a new MLOps infrastructure, and how it performs.
2021
1️⃣ Highly accurate protein structure prediction with AlphaFold -> (From DeepMind, 8965) AlphaFold, a breakthrough in protein structure prediction using Deep Learning. See also "Accurate prediction of protein structures and interactions using a three-track neural network" (from multiple academic institutions, 1659 citations), an open-source protein structure prediction algorithm.
2️⃣ Swin Transformer: Hierarchical Vision Transformer using Shifted Windows -> (From Microsoft, 4810 citations) A robust variant of Transformers for Vision.
3️⃣ Learning Transferable Visual Models From Natural Language Supervision -> (From OpenAI, 3204 citations) CLIP, image-text pairs at scale to learn joint image-text representations in a self-supervised fashion
4️⃣ On the Dangers of Stochastic Parrots: Can Language Models Be Too Big? -> (From U. Washington, Black in AI, The Aether, 1266 citations) Famous position paper is very critical of the trend of ever-growing language models, highlighting their limitations and dangers.
5️⃣ Emerging Properties in Self-Supervised Vision Transformers -> (From Meta, 1219 citations) DINO, showing how self-supervision on images led to the emergence of some sort of proto-object segmentation in Transformers.
2020
1️⃣ An Image is Worth 16x16 Words: Transformers for Image Recognition at Scale -> (From Google, 11914 citations) The first work showing how a plain Transformer could do great in Computer Vision.
2️⃣ Language Models are Few-Shot Learners -> (From OpenAI, 8070 citations) GPT-3, This paper does not need further explanation at this stage.
3️⃣ YOLOv4: Optimal Speed and Accuracy of Object Detection -> (From Academia Sinica, Taiwan, 8014 citations) Robust and fast object detection sells like hotcakes.
4️⃣ Exploring the Limits of Transfer Learning with a Unified Text-to-Text Transformer -> (From Google, 5906 citations) A rigorous study of transfer learning with Transformers, resulting in the famous T5.
5️⃣ Bootstrap your own latent: A new approach to self-supervised Learning -> (From DeepMind and Imperial College, 2873 citations) Showing that negatives are not even necessary for representation learning.
Read on below to see the full list of 100 papers for 2022, but let's first dive into the analyses for countries and institutions.
The most cited papers from the past 3 years
When we look at where these top-cited papers come from (Figure 1), we see that the United States continues to dominate and the difference among the major powers varies only slightly per year. Earlier reports that China may have overtaken the US in AI R&D seem to be highly exaggerated if we look at it from the perspective of citations. We also see an impact significantly above expectation from Singapore and Australia.
To properly assess the US dominance, let's look beyond paper count numbers. If we consider the accumulated citations by country instead, the difference looks even stronger. We have normalized by the total number of citations in a year, in order to be able to compare meaningfully across years.

Figure 2. Source: Zeta Alpha
The UK is clearly the strongest player outside of the US and China. However, the contribution of the UK is even more strongly dominated by DeepMind in 2022 (69% of the UK total), than in the previous years (60%). DeepMind has truly had a very productive 2022. Looking at the regions, North America is leading by a large margin while Asia is slightly above Europe.

Figure 3. Source: Zeta Alpha
Now let's look at how the leading organizations compare by number of papers in the top 100.

Figure 4. Source: Zeta Alpha
Google is consistently the strongest player followed by Meta, Microsoft, UC Berkeley, DeepMind and Stanford. While industry calls the shots in AI research these days, and single academic institutions don't produce as much impact, the tail for these institutions is much longer, so that when we aggregate by organization type, it evens out.

Figure 5. Source: Zeta Alpha
If we look into total research output, how many papers have organizations published in these past 3 years?

Figure 6. Source: Zeta Alpha
In total publication volume, Google is still in the lead, but differences are much less drastic compared to the citation top 100. You won't see OpenAI or DeepMind among the top 20 in the volume of publications. These institutions publish less but with higher impact. The following chart shows the rate at which organizations manage to convert their publications into top-100 papers.

Now we see that OpenAI is simply in a league of its own when it comes to turning publications into absolute blockbusters. While certainly, their marketing magic helps a lot to propel their popularity, it's undeniable that some of their recent research is of outstanding quality. With a lower paper volume but impressive conversion rate is also EleutherAI, the non-profit collective focusing on interpretability and alignment of large Language Models.
The top 100 most cited papers for 2022
And finally, here is our top-100 list itself, with titles, citation counts, and affiliations.
We have also added twitter mentions, which are sometimes seen as an early impact indicator, however the correlation so far seems to be weak. Further work is needed. Here you have the list for the year 2020 and for 2021 (as tsv files).
Methodology
To create the analysis above, we have first collected the most cited papers per year in the Zeta Alpha platform, and then manually checked the first publication date (usually an arXiv pre-print), so that we place papers in the right year. We supplemented this list by mining for highly cited AI papers on Semantic Scholar with its broader coverage and ability to sort by citation count. This mainly turns up additional papers from highly impactful closed-source publishers (e.g. Nature, Elsevier, Springer and other journals). We then take for each paper the number of citations on Google Scholar as the representative metric and sort the papers by this number to yield the top-100 for a year. For these papers we used GPT-3 to extract the authors, their affiliations, and their country and manually checked these results (if the country was not clearly visible from the publication, we take the country of the organization’s headquarters). A paper with authors from multiple affiliations counts once for each of the affiliations.
Updates 2023/03/07
- Update the 2022 list with the following papers:
- Emergent Abilities of Large Language Models (74 citations)
- DeiT III: Revenge of the ViT (44 citations)
- Fix missing EleutherAI as the 2nd best organization in terms of conversion rate
- Added links to the full 2020 top-cited paper list and 2021 top-cited paper lists
- Add counts by region plot
- Fix missing countries and organizations in the 2022 list
This concludes our analysis; what surprised you the most about these numbers? Try out our platform, follow us on Twitter @zetavector and let us know if you have any feedback or would like to receive a more detailed analysis for your domain or organization.